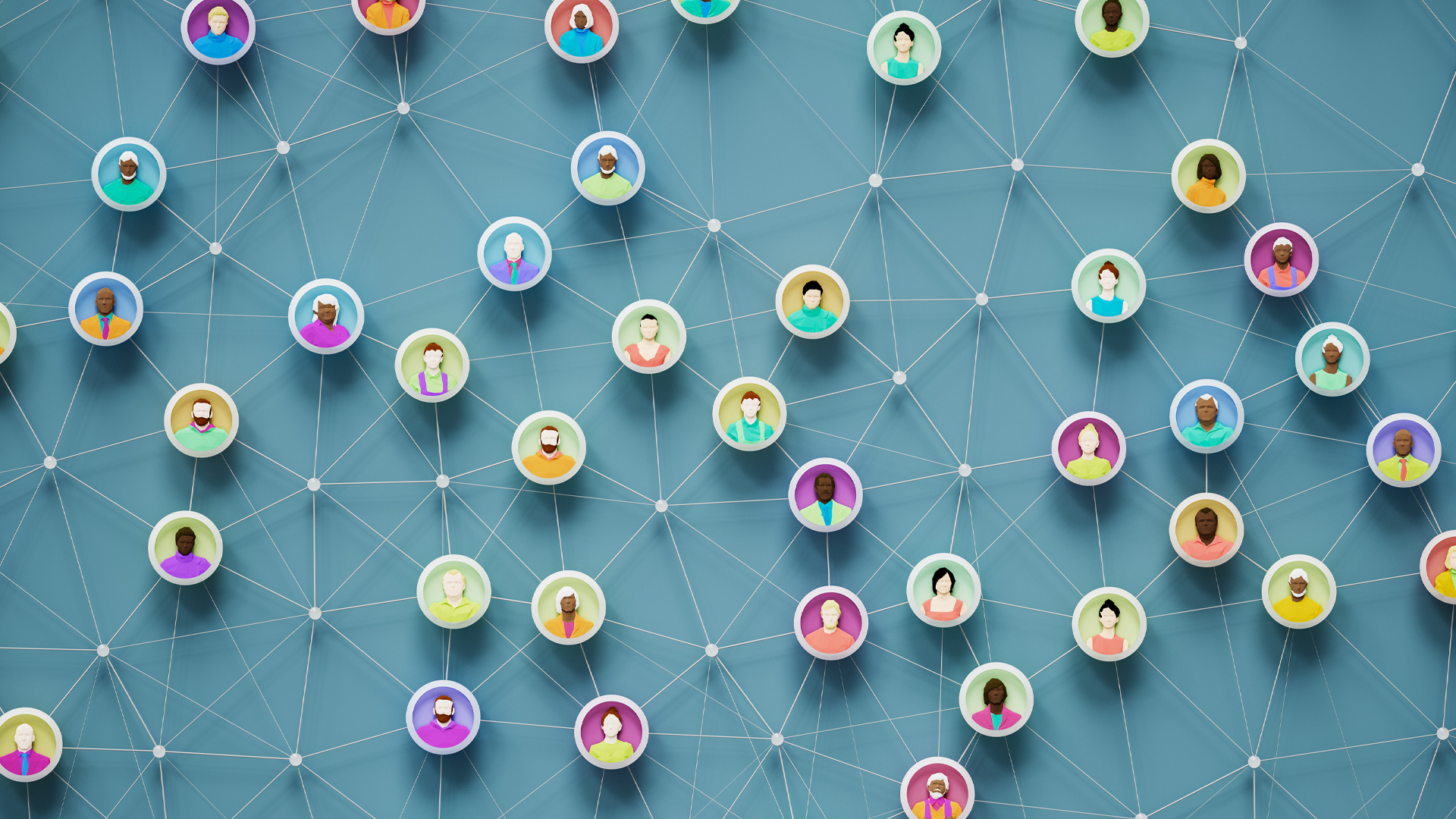
New technologies follow a fairly predictable cycle: First comes the hype, then disappointment, followed finally by measured and thoughtful acceptance. Artificial intelligence (AI) is still largely in the hype phase, still a novelty with a magical veneer.
But we are on the cusp of moving past hype, and we need to start looking for new governance approaches to address this emergent technology.
If projections of market size are any indication, AI is about to be everywhere. Making headway today on AI governance will help to ensure that governments do not fall farther behind when the rubber really starts to hit the road.
One way to get the ball rolling on rules for AI is a “negative list” approach, the concept of limiting AI with explicit prohibitions – “red lines” or no-go zones – that will help provide a playbook for rule-making in AI so as to ensure that the public interest is considered as AI becomes increasingly ubiquitous.
A negative list approach might not satisfy the desire among policy wonks to roll out a comprehensive policy solution that will stand the test of time, but it does represent an immediate pathway to much-needed progress in AI governance.
But what exactly is in the public interest when we talk about AI?
It is a challenging question. Defining and safeguarding the public interest in this area is complicated because the implications of the technology are vast and pervasive. One need only consider the broad impact of search engines or precision digital advertising (both AI applications) to get a sense of how disruptive AI might be as it becomes increasingly ubiquitous. The growing pervasiveness of AI applications is also a significant challenge in terms of how governments can align their policy actions with citizens’ expectations because social values surrounding new technologies are hard to measure with certainty.
Certainly, public-opinion data does exist, but opinions about developments like AI are not set in stone. They are likely to oscillate drastically depending on levels of confusion and misconceptions as time goes on. One need only consider how social media radically transformed public expectations and understanding of privacy in only a few years to get a sense of how public opinion about disruptive technologies can be unpredictable.
This makes the standard policymaking toolkit harder to utilize. Indeed, how do we govern something whose application cannot be easily defined and that the public values inconsistently?
It is useful to focus less on applications receiving signals of public approval and more on signals of public disapproval. Behavioural economics suggests that individuals and the public at large tend to seek to avoid a loss more vigorously than they pursue a gain of equal value.
In this sense, firm opposition to AI applications could prove to be a much better signpost for policy wonks looking to improve AI governance than would indications of tepid agreement, which cannot be interpreted as social license.
Take, for example, the famous Target case, where the retailer used data science and AI to predict likely shopping habits, and also ended up correctly predicting one of its teenage shoppers was pregnant, which came as a surprise to her family. Target hadn’t done anything illegal, but these activities didn’t inspire public confidence, either. The company, realizing that it was running against acceptable norms, adjusted its strategy accordingly.
The expert discourse around AI is not sufficiently mature to allow for a coherent positive vision for AI regulation to be developed anytime soon, but a negative vision for AI rule-making is within grasp, and would provide a path forward, leading eventually to a comprehensive list of AI “don’ts,” which is still a long way off.
We could start right now by setting explicit limits for situations that are obviously problematic: war, for example. There have been calls for AI to be banned in war, with an end to the development of autonomous weapons systems. Limits are also needed on the use of AI that targets children and the elderly.
We don’t need to start from a blank slate. Here is where public opinion in other circumstances can be used as a guideline. If, say, guerilla marketing targeting the elderly is generally frowned upon, AI-backed data mining and analysis will likely be frowned upon as well. Not only is strong disapproval easier to measure than shades of grey, it is much more predictable than more nuanced opinions, even for a subject as ambiguous as AI. It is well within government’s capacity to start to anticipate some no-go zones and to implement policy proactively.
Important but manageable conversations need to be had by policy wonks on the question of when it is not in the public interest to exploit the full technical potential of AI.
Putting particular limits on AI – the negative-list approach – is a manageable way of making rules. It offers a clear roadmap for safeguarding the public interest, and it could demystify AI by using precedents and building on lessons learned from comparable circumstances.
Rather than jumping into the deep end of AI regulation, policymakers should start now by gradually wading in with discussions about how existing legal and policy frameworks should be interpreted within AI-specific contexts.
Photo: Shutterstock by Who is Danny
Do you have something to say about the article you just read? Be part of the Policy Options discussion, and send in your own submission. Here is a link on how to do it. | Souhaitez-vous réagir à cet article ? Joignez-vous aux débats d’Options politiques et soumettez-nous votre texte en suivant ces directives.